AI Is Making Data Centers Thirsty
The artificial intelligence boom isn't only putting pressure on data centers' power usage, it's also forcing them to consume millions of gallons of additional water.
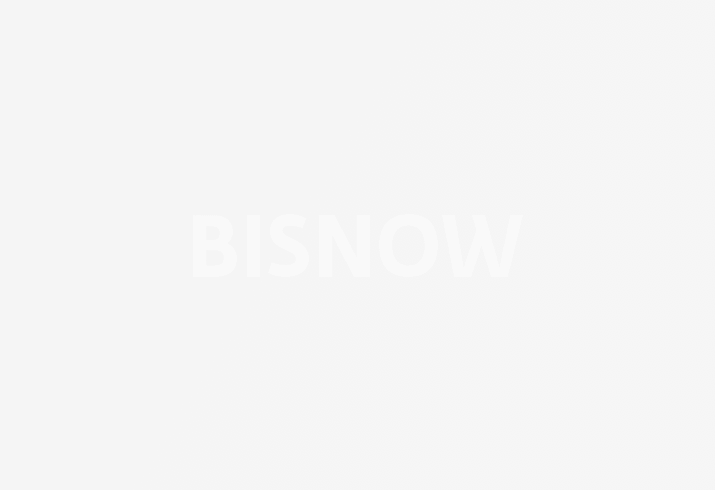
The computing power behind generative AI technologies like ChatGPT and Google’s Bard is helping drive up the data center industry’s already-significant water usage. Just five queries to ChatGPT can result in the consumption of around 16 ounces of water, according to a study from the University of California, Riverside, the same as a standard bottle of Dasani.
But even as AI becomes a larger piece of the data center ecosystem, experts say there is a realistic path to tempering the industry’s growing thirst and the potential impact on water-sensitive regions.
“This is something organizations have to think about now. They realize that you can’t just completely greenwash and forget to be efficient,” said Bill Kleyman, a longtime data center executive who sits on the board of AI infrastructure startup Neu.ro. “There are some good options out there to be efficient.”
Even before the advent of generative AI, data centers’ massive water consumption was one of the most prominent sustainability challenges facing the industry.
Data halls filled with thousands of heat-producing processors and network equipment need to be cooled. That can be done in a number of ways, but the process typically involves so-called evaporative cooling that uses millions of gallons of water in all but a few of the newest data centers. In 2022, Google consumed more than 5.2 billion gallons of water to cool its self-operated data centers — a total the company estimates as the equivalent of operating 34 golf courses during the same period.
The IT equipment supporting generative AI often necessitates even higher water consumption compared to the traditional servers that have been housed in data centers for years. This is because the high-performance servers used for AI computing use significantly more power and thus produce significantly more heat.
“Because AI generally has a much higher energy density, it would need more cooling for the same space,” said Shaolei Ren, a professor of electrical and computer engineering at UC Riverside and the lead author of the study. “If you consider the broader water footprint, it’s even higher because generating electricity requires water.”
Ren said the water used by the power plants producing the megawatts of energy AI requires should be included when evaluating AI’s water consumption.
According to Kleyman, while the electricity needed for a standard Google search could power a 100-watt light bulb for 11 seconds, the power used by ChatGPT to answer a single question could charge a cellphone more than a dozen times. Today, most of this power is coming from nonrenewable energy sources that themselves consume massive amounts of water for cooling.
This off-site water footprint often exceeds the water used at the data center itself. According to the UC Riverside study, while training ChatGPT-3 in Microsoft’s data centers directly consumed 700,000 liters of freshwater, it was responsible for a 2.8-million-liter off-site water footprint from its power consumption.
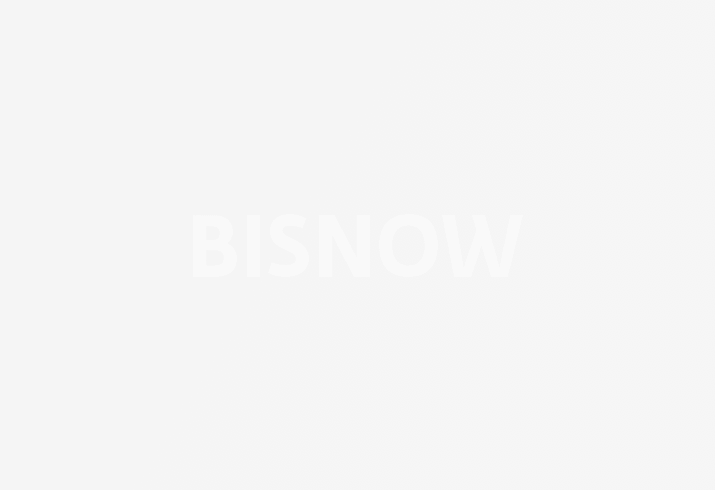
While generative AI currently accounts for a small fraction of data center workloads, the technology is in its infancy. AI’s role within the data center space is expected to grow dramatically, with hyperscalers already building and leasing new capacity as fast as possible to support AI applications that are increasingly central to their business.
Although there is no reliable data on what share of the data center industry’s overall water use can be attributed specifically to AI applications, Ren said they play a disproportionate and rising role in usage numbers reported by tech giants like Microsoft and Google.
“I think generative AI is certainly a big contributor,” Ren said. “The growth rate of generative AI is definitely higher than the other regular services they had in the past.”
But even amid this growth, an AI-driven water crisis isn't an inevitability, industry experts said. While most stressed the need for greater urgency, some Big Tech hyperscalers and third-party data center providers have taken initial steps toward more efficient water use for AI computing.
At the Des Moines, Iowa, data center campus where Microsoft trained ChatGPT, water use has plummeted, with its usage from January through July down 20% year-over-year and down 43% from the same months in 2021, according to data from the local water utility. This vast improvement in efficiency was likely the result of a simple change, according to Ren: adjusting operational guidelines to increase the temperature at which evaporative cooling is used.
Microsoft declined to answer questions about its Iowa data center, but the firm provided a statement to Bisnow on its efficiency efforts.
"As part of our commitment to create a more sustainable future, Microsoft is investing in research to measure the energy use and carbon impact of AI while working on ways to make large systems more efficient, in both training and application," Microsoft's statement says. "We will continue to monitor our emissions, accelerate progress while increasing our use of clean energy to power datacenters, purchasing renewable energy, and other efforts to meet our sustainability goals of being carbon negative, water positive and zero waste by 2030."
Elsewhere, third-party data center providers like Nautilus Data Technologies are taking innovative approaches to cooling to improve water efficiency, from floating data centers and facilities that use naturally cold water from rivers and oceans to the utilization of waste “grey water.”
While these scattered approaches offer potential tools for mitigating rising water usage, the authors of the UC Riverside study argue that meaningfully mitigating water consumption will require hyperscalers, third-party data center providers and their customers to prioritize sustainability when it comes to both where and when they run the most energy-intensive AI workloads.
This means avoiding training AI models in places like Phoenix, where water resources are stretched thin and where high temperatures necessitate more water-intensive cooling than in colder climates. Because latency and network connectivity are of little importance for AI training, the massive computing tasks can be run in locations with efficient cooling and fueled by clean energy.
“It's compute-intensive, but it's not latency-sensitive, so you can absolutely train it in the middle of Idaho or Nebraska and be super efficient,” Kleyman said. “That's something that we're absolutely doing.”
It’s not just location: Timing matters as well. Experts said companies training AI models could significantly lower the amount of water needed for cooling by only running training workloads during colder months or at night. Off-site water consumption can also be limited by avoiding training during periods of high energy demand, when power grids typically have a lower percentage of power from renewable sources.
“For electricity generation, the fuel mix changes depending on demand,” Ren said. “If there’s a higher mixture of thermal or nuclear or hydropower, the water consumption for generation will probably be higher as well.”
Neu.ro has employed this concept, according to Kleyman. The company’s platform offers a “green scheduler” that helps users locate and time workloads based on sustainability criteria. Google has also experimented with this approach on a small scale, implementing a Carbon-Intelligent Compute Management pilot program that adjusted the scheduling of flexible tasks like AI training based on sustainability factors.
But while these tools exist, they aren't widespread. That may have to change as the AI arms race continues.
“I think in the next five to 10 years, we're going see more adoption of these technologies into more of these bigger companies,” Ren said.