‘Don’t Move Too Fast’: Why An ‘Intensely Practical’ Approach To CRE Data Is Needed In Today’s Market
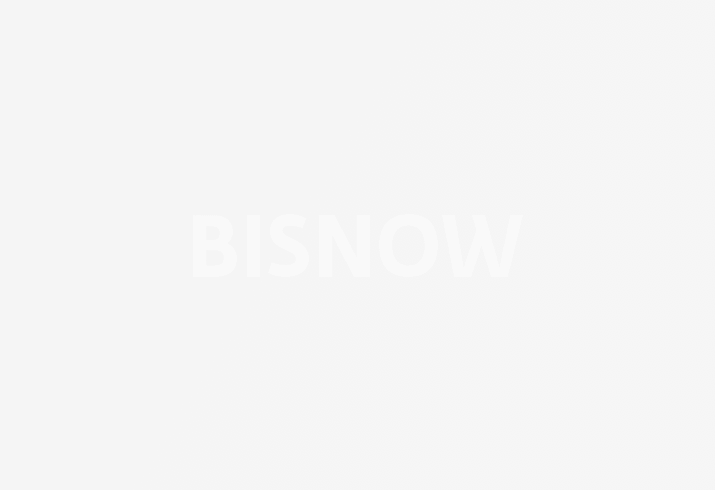
It is evident that the world sits on the cusp of an artificial intelligence boom. The AI/machine learning industry is expected to grow by nearly 40% this year alone. The sector is anticipated to produce $16T of revenue globally by 2030, more than 300 times greater than the $51B it generated in 2022.
Commercial real estate relies heavily on data accuracy to make informed investment decisions. Whether it is used to mitigate investment risks, evaluate pricing models or improve tenant experiences, analytics are essential to the industry, but the recent use of machine learning and artificial intelligence in commercial real estate data is widely regarded as game-changing.
While the integration of machine learning tools into daily CRE business operations is expected to bring new innovations to the industry, the challenge becomes how CRE firms can maintain focus on achieving their key performance indicators and develop the best tools for the task among the escalating number of options and approaches in the market.
“Working with data in CRE is nothing new, but what is new is the data science tools that are available today,” said Mike Sobolik, North American strategist at Invesco Real Estate. “For every useful data solution there are thousands of bright, shiny objects that can distract a data team and an executive team. What you really have to keep in mind is your endgame.”
Sobolik said in the fast-moving world of data science, the best technology is probably different from what it was two years ago, especially in the CRE world, which hasn't traditionally been the most technologically inclined industry. As new machine learning and AI tools are produced, it is best to pace their introduction to the sector, creating an “intensely practical” approach to real estate data, he said.
“It's important that the purpose and goals of the business drive development of technology tools, and not the other way around,” Sobolik said. “Everything you do must be guided by your North Star, or how success is defined for your organization.”
For an investment management firm like Invesco, the North Star is improved investment performance. However, North Stars that guide other types of CRE businesses, such as brokerages or development firms, will most likely look different depending on the purpose or mission of the firm and the clients it serves. Key performance indicators can then be used to guide the firm toward achieving its overall objectives.
Sobolik said data projects should be designed to serve and feed into the firm's main purpose, and other data projects that don't serve this purpose other than a dedicated research and development discipline can be a distraction.
A “no silos” data environment where collaboration between data scientists and business practitioners happens regularly is also key to creating an intensely practical approach to data in CRE, said Nicholas Buss, managing director of strategic analytics at Invesco Real Estate. Data initiatives can't be exercises done at arm’s length — everyone in the company must be on the same page for data tools to be effective and actionable.
“CRE firms need to define goals together, scope projects together and creatively iterate back and forth until a highly usable solution is reached,” Buss said. “If there’s no communication, a data science team can create a set of tools that make sense to them, but if it doesn't make sense to the actual users, then you've wasted a lot of resources.”
Buss said it takes not only effective and frequent communication to create a “no silos” environment, but also mutual respect.
While one team may be proficient in data and analytics, another may be more inclined toward sales or operations — rendering it beneficial for all parties to listen and acknowledge each other’s ideas. Data scientists often call this “keeping humans in the loop” so feedback can be given that will overall improve the success and effectiveness of a data model.
“Data scientists must be excellent technicians as well as well-rounded athletes,” Buss said. “They must bring a high degree of creativity to their work, but also should be able to collaborate well with others in the field, including investment practitioners and C-suite executives.”
Sobolik said that while KPIs help make data usable, making it digestible is a different story.
Executive teams focus on the big picture, so data scientists need to distill their work to some simple and visual decision metrics rather than weighing down the process with excessive technical output, he said.
“CRE data models are trying to show cause-and-effect relationships based on what's happened in the past,” Sobolik said. “You have to understand the periods of time that the data represents to get an idea as to how well it can depict a future outcome based on what's happened already.”
Every model is going to have limitations because each business cycle is different, and human judgment will always need to come into play, Sobolik said. At Invesco Real Estate, CRE data tools are put into a more probabilistic framework as opposed to forecasts. The data science team is focused on getting relativity correct rather than the magnitude of what is being measured. The team estimates a range of outcomes instead of just one path.
“Our investment performance is measured against a benchmark, so we're looking for what can drive that performance relative to that benchmark and understanding how markets stack up against each other,” Buss said. “We view the analytics that we put together as just one piece of the puzzle, and we use it to drive an intensely practical approach to our business.”
This article was produced in collaboration between Invesco and Studio B. Bisnow news staff was not involved in the production of this content.
Studio B is Bisnow’s in-house content and design studio. To learn more about how Studio B can help your team, reach out to studio@bisnow.com.