ChatGPT Is Just The Start: AI Is Rewriting The Data Center Real Estate Map
Artificial intelligence is finally having its moment, and it is dramatically transforming the data center real estate landscape.
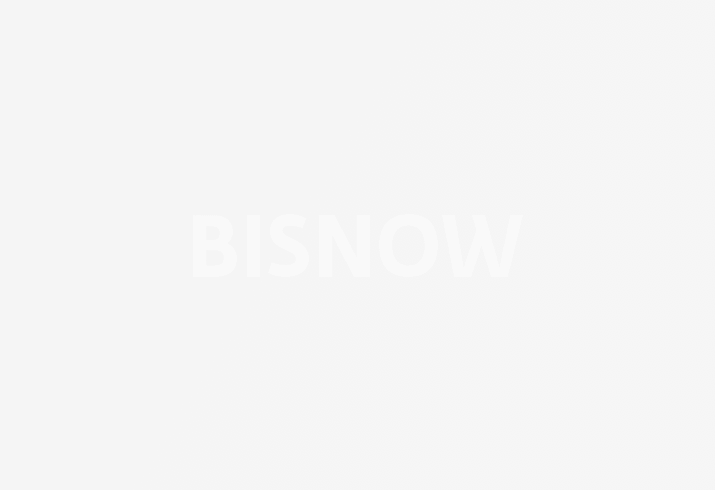
ChatGPT — the web-based AI interface that responds to user questions with often strikingly human written responses — has captured the public imagination since its release by OpenAI in November.
Indeed, access to a technology that can instantly create both real estate marketing emails and a biblical verse explaining how to remove a peanut butter sandwich from a VCR seems like a massive leap toward a future envisioned in science fiction novels.
While “generative” AI like ChatGPT and OpenAI’s image-creating DALL-E may be little more than high-tech toys in their current forms, they are the leading edge of a wave of AI-centered digital transformation that data center industry leaders say is already well underway. The widespread adoption of AI may be the most significant force reshaping the digital infrastructure map in the months and years ahead, transforming both where and how data centers are built.
“You look back, the last 20 or 30 years, data centers were built out around the needs of the video streaming applications driving demand, that’s what all the infrastructure was built for,” said Wes Cummins, CEO of specialty data center provider Applied Digital. “The apps driving demand going forward are going to be around artificial intelligence. This kind of compute is going to be where the demand will be for the next 10 or 20 years.”
The world’s largest tech companies are betting their digital infrastructure dollars on an AI-driven future.
Earlier this month, Microsoft announced a $10B investment in OpenAI for the continued evolution and expansion of ChatGPT, on top of a previous $1B investment in the company. Google has also made big investments in AI development, while Meta last month paused data center build-outs around the world in order to redesign them to be optimized for AI applications.
Industry insiders say the past few months have been a key inflection point for AI innovation, with the adoption curve for these technologies poised to get much steeper in the near future.
Developments like the addition of OpenAI’s tools and services to Microsoft’s Azure platform are lowering cost barriers to creating new AI products. Experts say this is driving a wave on innovation and creating demand for the massive amounts of computing power needed to build and operate these technologies.
“You’re going to see a lot of applications, and from a venture standpoint you’re already seeing this new landscape evolve in terms of these more generative-type technologies,” said Ari Kamlani, senior AI technology strategist and systems architect at Beyond Limits, a developer of commercial AI applications. “There’s an explosion of the generative landscape, as well as in how people interact with these technologies.”
More AI means a growing need for computing power, and that means more data centers. But the equipment and infrastructure required for most AI applications differs significantly from the servers most data center providers have been hosting for decades.
In general, AI requires faster, more powerful processors than those found in most traditional data center servers. Indeed, it has been the development of these high-performance chips that has largely enabled this wave of AI innovation. But while these so-called GPU processors are better at performing the required calculations quickly, they use much more power and create significantly more heat than the equipment for which most data centers are designed.
As a result, many providers are already changing their facility designs to get out in front of the growing wave of demand for AI computing capacity.
According to Flexential Chief Innovation Officer Jason Carolan, this means redesigning everything from backup power systems and generators to liquid-based cooling systems and changing building locations to be closer to nearby substations.
“It’s starting to really change design requirements for the next-generation data center,” Carolan said.
AI is not only changing how data centers are built, it’s also changing where they’re built.
Among the data center experts who spoke with Bisnow, there was a consensus: widespread adoption of AI will accelerate the decentralization of the data center landscape, driving development in new markets and away from the industry’s traditional hubs like Silicon Valley and Northern Virginia.
One significant factor is the massive power requirements for AI computing. Rising energy costs and shortages of power in the industry’s traditional hubs are already driving data centers into new territories, with developers increasingly prioritizing locations where they have access to cheap and ideally renewable electricity. AI may well accelerate this trend as its adoption increases how much power each facility needs.
At the same time, experts say driving down the price of compute for AI through lower power costs is imperative if AI applications are going to be financially viable. At present, the enormous cost to access needed computing power through the cloud or from a data center directly is broadly prohibitive. ChatGPT operates at a significant loss, spending over $100K daily on compute alone.
But AI’s transformation of the data center landscape is more complicated than just the quest for cheap power. Experts say the distinct architecture of AI systems will create two distinct demand vehicles for data center space.
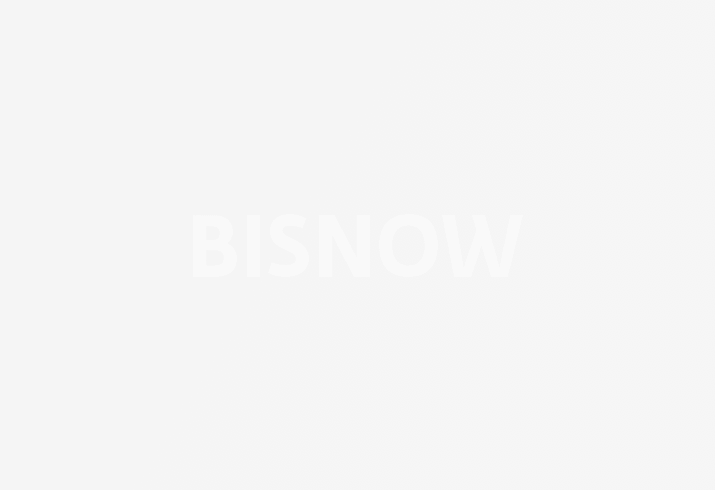
The interconnected computing systems making up an AI application can generally be divided into two parts with different infrastructure requirements — effectively two hemispheres of a single brain.
In one part, a massive amount of computing power is used for what is called “training”: giving an AI model access to a massive amount of information — in the case of ChatGPT, the whole internet — from which it can develop a decision-making framework that can be applied elsewhere.
Once this decision-making framework has been created, it can be run on a different set of infrastructure for users to interact with in real time. This latter stage, where the AI is actually applied, is known as “inference.”
“You’re taking 300 billion data points, you crunch them, and you train a model that then makes decisions based on all the data points, and then you constantly refine that model,” Applied Digital’s Cummins said. “You train the model in one environment, and then you can upload that model into a real-time operating environment for the decision-making."
The need for data center space to host both of these computing environments is reshaping the data center map, experts say, but in very different ways.
AI training — requiring larger amounts of computational power but little need for lightning-fast connectivity with end users or other facilities — will drive demand in markets with low power costs and renewable energy, even if they don’t have the kind of connections to fiber networks that would be required in a traditional data center, experts say.
Applied Digital specializes in exactly this kind of facility, with its main AI-focused facility under development in North Dakota. The primary consideration, Applied's Cummins said, is providing the lowest possible cost of compute. While this primarily means cheap power, costs can also be kept down by building facilities without the redundancies needed in traditional mission critical data centers, and by locating them in cold locations where less power has to be used on cooling.
“Most data centers are kind of Swiss Army knives — they were built for everything, whether you're running video, Microsoft apps for your business or whatever it might be,” Cummins said. “We're building it specifically for these use cases, so it makes it much cheaper.”
While AI training is driving demand for data centers in far-flung markets, the need for computing power to deploy these technologies for end users is helping drive data center demand in close proximity to major population and business centers at what the data center industry calls “the edge.”
Flexential’s Carolan points to AI use cases like self-driving cars or a customer service call center that uses language processing AI in real time. That kind of application requires a ton of computing for inference, and it needs to happen almost instantly. Achieving that kind of latency requires placing that computing capacity — and the data centers it lives in — near the end user. It also means building out the dense fiber networks needed to make sure data can be moved quickly.
Carolan said hyperscalers are already making significant investments in both edge AI computing infrastructure and the networks needed to support them. He said the creation of these fiber access points mean other data center operators follow close behind, as AI leads to the creation of new mini digital infrastructure hubs away from traditional locations like Northern Virginia.
“It’s growing the data center business not only because the hyperscalers want to be in new regions, but that’s also causing other data centers to be close to the hyperscalers for the connectivity,” Carolan said. “There’s this growing architecture of edge to medium to large processing centers where they have a tendency to attract other data centers around them, because people want to be close to those facilities, largely because they want a super-fast pipe between their infrastructure in the cloud to be able to move processing around.”